NFTs have been on the rise for the past few years. What was once a small part of the blockchain world has become a huge market for digital art, collectibles. Virtual real estateand most of the NFTs have been sold at crazy prices, others quickly disappear into thin air. In this rollercoaster of an environment, more and more creators and investors are turning to predictive analytics to understand what the future holds for NFT valuations. But can AI really predict the next big thing in NFTs?
Below we'll look at how predictive analytics works, the data points in NFT valuations, the AI tools used to interpret those data points, and where the market is headed in the near future.
Why are data-driven insights important in the NFT market?
Simply put, predictive analytics uses historical data and advanced algorithms to identify patterns, predict outcomes, and guide decision making. When applied to NFTs it means collecting and Data analysis such as past sales, social media chatter and market sentiment to predict the future performance of an NFT or an entire class of NFTs.
NFTs have attracted the attention of analysts, venture capitalists and even large corporations. While some still refuse Digital collectionsOthers consider these signs to be the foundation Web 3. As the market grows, understanding pricing patterns is key for creators who want to price their work fairly and for investors who want to find undervalued jewelry.
Basics of predictive analytics
Predictive analytics relies on several key components:
Data collection: Collecting a wide variety of data—NFT transaction records, social media posts, on-chain analytics, etc.—is critical.
Model selection: Different models are suitable for different problems. Whether it's a time series or a neural network, the choice can make a big difference.
Engineering Feature: This step involves converting the raw data into features. For example, the level of rarity of an NFT may be considered as a numerical value or even an emotional score from social media.
Correlation vs Causation: It is easy to confuse correlation with causation. For example, an NFT price increase may coincide with a celebrity tweet, but that does not mean that the tweet caused the price increase.
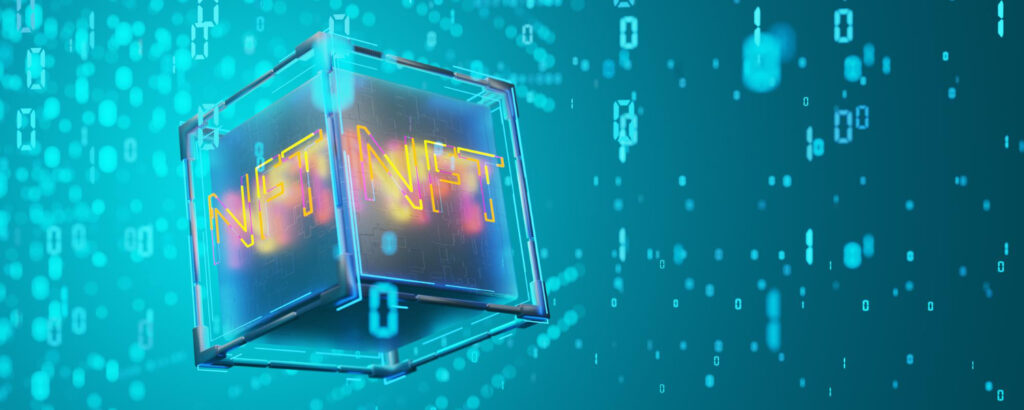
Data points for NFT valuation models
On-chain data
One of the biggest selling points of NFTs is transparency. Anyone can view blockchain records for sales history, wallet addresses, and transaction timing. These data points help analysts see demand patterns. If a certain set has new wallets every week, it may be a sign of an upward movement in price.
Social media sentiment
Twitter and Discord are the meeting places for NFT enthusiasts. Analyzing users' mentions, hashtags, and sentiment can reveal emerging promotional cycles or highlight projects with strong communities. AI-powered sentiment tools can scan thousands of messages to see the overall sentiment surrounding a particular NFT project.
The reputation of the creator or brand
Famous manufacturers or brands attract more attention NFT markets. Artists with a history of successful falls or a strong background in traditional art may increase the value of their NFT. AI can track past performance data along with brand mentions and see how a manufacturer's reputation correlates with pricing.
Broader crypto market factors
NFTs do not exist in isolation. Especially crypto markets Ethereum and Solana It can affect NFT values. High gas costs or negative sentiment towards cryptocurrencies in general can scare off buyers. Conversely, bullish trends in major coins can spill over and bring new buyers into NFTs.
Time series analysis
Time series models –Arima or Advanced Recurrent Neural Networks– Can be used to predict how the price or trading volume of NFTs will change over days or weeks. They are good at recognizing cycles but struggle with sudden changes caused by viral social media chatter.
Machine learning regressions
Linear regression or gradient boosting Machine learning models It can take multiple input attributes—social media mentions, trading volume, etc.—and generate a predicted price. The success of these models depends on the amount and quality of data.
Neural networks for pattern recognition
Deep learning algorithms It can find patterns in large data sets that are missed by traditional methods. For example, a neural network might detect early changes in sentiment based on how people talk about a project rather than the number of positive or negative words.
Automated dashboards
Nansen or DappRadar offer analytics dashboards that collect blockchain data, track wallet movements, and visualize trending collections. While these tools are powerful, they are only as good Data and algorithms they use
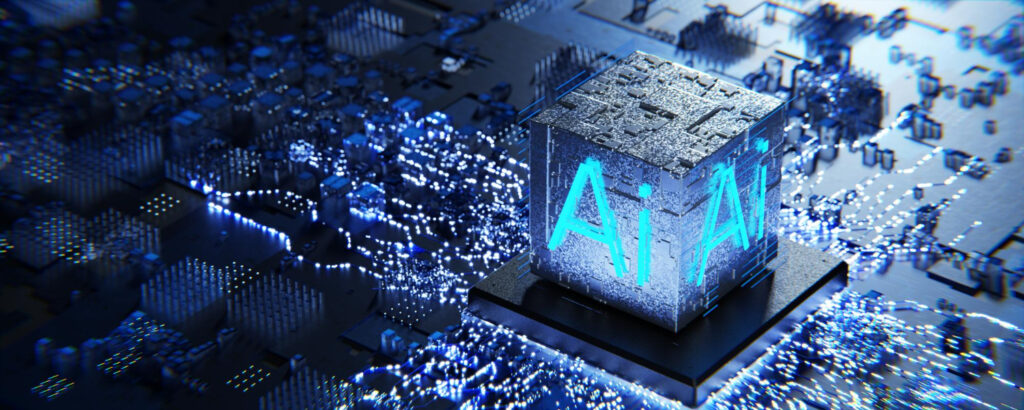
Potential problems and challenges
Data quality and availability
NFTs are recorded on the ledger but each market has different data reporting standards. Inconsistent or incomplete data can confuse AI models. Analysts must review sources and possibly combine data from multiple platforms.
Fast moving trends
NFTs can follow meme-based hype cycles that appear and disappear within weeks, if not days. AI models trained on older data may miss these rapid changes, especially if they are based on historical patterns that no longer apply.
Market Manipulation (Wash Trading)
Some NFT makers or holders may launder the trade, artificially inflating sales numbers to create the illusion of demand. It can easily skew chain data and mislead AI models.
Limitations of the numerical approach
Not everything about NFTs can be reduced to price charts and volume metrics. Community spirit, developer reputation and even cultural relevance can make a big difference. Relying too much on numbers can miss intangible variables that affect long-term value.
Future vision
Experts expect NFT space grow, but the market may move from speculation to functional tokens such as game assets or membership tokens. As the market evolves, AI gets better at understanding these changes. Meanwhile, the convergence of NFTs, metaverse and new blockchain protocols opens up new data analytics and Predictive modeling Opportunities
In addition, institutional investors will begin to pay attention to NFT analysis and apply the same data-driven methods as traditional finance. This leads to more mature markets with standardized practices and ultimately more reliable predictive analytics.
Final thoughts
While predictive analytics and AI are great at finding patterns, they are not infallible. The NFT world is all about innovation, community and viral content – things that can't be measured by a set of numbers. But combining the power of artificial intelligence with human intuition and a sense of the cultural space of the market can help collectors and creators make better decisions.
As NFTs move out of the hype cycle and into practical use cases, the demand for analytics will increase. Whether you're an artist looking for fair pricing for your work or an investor looking for early-stage projects, paying attention to AI-powered insights while acknowledging the limitations of machine-based prediction puts you in the best position to succeed in this wild and crazy environment. puts .
Editor's note: This article was written with the help of artificial intelligence. Edited and reviewed by Owen Skelton.